Global research on use of artificial intelligence in imaging for breast cancer detection: bibliometric analysis
Investigación global sobre uso de inteligencia artificial en imagenología para la detección de cáncer de mama: análisis bibliométrico | 全球乳腺癌检测影像学中人工智能应用的研究:文献计量分析
DOI:
https://doi.org/10.25176/RFMH.v24i3.6407Keywords:
Artificial Intelligence, Mammography, Mammary Ultrasonography, Breast Neoplasms, BibliometricsAbstract
Introduction: Breast cancer remains one of the most prevalent cancers globally, specifically the most common in females. The use of artificial intelligence promises to contribute to early diagnosis through imaging. Previously, the landscape and evolution of this scientific production have not been described.
Methods: Cross-sectional bibliometric study using Scopus as the data source. The bibliometrix package in R was employed for calculating bibliometric indicators and visualizing the results.
Results: 1292 documents published between 1989 and 2024 were selected. 75.3% (n=973) were articles with primary data, followed by 16.2% (n=209) corresponding to reviews. An international collaboration rate of 26.5% was identified, with an annual production growth of 10.78%. It was observed that risk classification through screening, digital breast tomosynthesis, transfer learning, segmentation, and feature selection were the most commonly used keywords. In the last five years, deep learning and mammography have been the most popular topics. International collaboration has been led by the United States, China, and the United Kingdom.
Conclusions: A notable growth in global research on the use of artificial intelligence in breast cancer imaging for detection was identified, particularly since the 2010s, primarily through the publication of articles with primary data. The relationship between artificial intelligence and imaging for breast cancer diagnosis has focused on risk and prediction.
Downloads
References
World Health Organization. Breast cancer [Internet]. [Consultado 10 Febrero 2024]. Disponible en: https://www.who.int/news-room/fact-sheets/detail/breast-cancer
Pan American Health Organization. Cáncer de mama [Internet]. [Consultado 10 Febrero 2024]. Disponible en: https://www.paho.org/es/temas/cancer-mama
Martei YM, Pace LE, Brock JE, Shulman LN. Breast Cancer in Low- and Middle-Income Countries: Why We Need Pathology Capability to Solve This Challenge. Clin Lab Med. 2018; 38(1):161-173. doi: 10.1016/j.cll.2017.10.013
Francies FZ, Hull R, Khanyile R, Dlamini Z. Breast cancer in low-middle income countries: abnormality in splicing and lack of targeted treatment options. Am J Cancer Res. 2020; 10(5):1568-1591.
Reyes A, Torregrosa L, Lozada-Martinez ID, Cabrera-Vargas LF, Nunez-Ordonez N, Martínez Ibata TF. Breast cancer mortality research in Latin America: A gap needed to be filled. Am J Surg. 2023; 225(5):937-938. doi: 10.1016/j.amjsurg.2023.01.010
Reyes-Monasterio A, Lozada-Martinez ID, Cabrera-Vargas LF, Narvaez-Rojas AR. Breast cancer care in Latin America: The ghost burden of a pandemic outbreak. Int J Surg. 2022; 104:106784. doi: 10.1016/j.ijsu.2022.106784
Gerami R, Sadeghi Joni S, Akhondi N, Etemadi A, Fosouli M, Eghbal AF, et al. A literature review on the imaging methods for breast cancer. Int J Physiol Pathophysiol Pharmacol. 2022; 14(3):171-176.
Aristokli N, Polycarpou I, Themistocleous SC, Sophocleous D, Mamais I. Comparison of the diagnostic performance of Magnetic Resonance Imaging (MRI), ultrasound and mammography for detection of breast cancer based on tumor type, breast density and patient's history: A review. Radiography (Lond). 2022; 28(3):848-856. doi: 10.1016/j.radi.2022.01.006
Ahn JS, Shin S, Yang SA, Park EK, Kim KH, Cho SI, et al. Artificial Intelligence in Breast Cancer Diagnosis and Personalized Medicine. J Breast Cancer. 2023; 26(5):405-435. doi: 10.4048/jbc.2023.26.e45
Meng G, Xu H, Yang S, Chen F, Wang W, Hu F, et al. Bibliometric analysis of worldwide research trends on breast cancer about inflammation. Front Oncol. 2023; 13:1166690. doi: 10.3389/fonc.2023.1166690
Teles RHG, Hiroki CT, Freitas VM. Bibliometric analysis of an important diagnostic technique for the treatment of breast cancer. Transl Cancer Res. 2022; 11(10):3440-3442. doi: 10.21037/tcr-22-2120
Xu J, Yu C, Zeng X, Tang W, Xu S, Tang L, et al. Visualization of breast cancer-related protein synthesis from the perspective of bibliometric analysis. Eur J Med Res. 2023; 28(1):461. doi: 10.1186/s40001-023-01364-4
Lozada-Martinez ID, Lozada-Martinez LM, Fiorillo-Moreno O. Leiden manifesto and evidence-based research: Are the appropriate standards being used for the correct evaluation of pluralism, gaps and relevance in medical research? J R Coll Physicians Edinb. 2024. Online ahead of print. doi: 10.1177/1478271524122799
Lozada-Martinez ID, Visconti-Lopez FJ, Marrugo-Ortiz AC, Ealo-Cardona CI, Camacho-Pérez D, Picón-Jaimes YA. Research and Publication Trends in Pediatric Surgery in Latin America: A Bibliometric and Visual Analysis from 2012 to 2021. J Pediatr Surg. 2023; 58(10):2012-2019. doi: 10.1016/j.jpedsurg.2023.04.003
Lozada-Martinez ID, Lozada-Martinez LM, Cabarcas-Martinez A, Ruiz-Gutierrez FK, Aristizabal Vanegas JG, Amorocho Lozada KJ, et al. Historical evolution of cancer genomics research in Latin America: a comprehensive visual and bibliometric analysis until 2023. Front Genet. 2024; 15:1327243. doi: 10.3389/fgene.2024.1327243
Aria M, Cuccurullo C. Bibliometrix: An R-Tool for Comprehensive Science Mapping Analysis. J Informetr. 2017; 11:959–975.
Rousseau R, Egghe L, Guns R. Becoming Metric-Wise: A Bibliometric Guide for Researchers. 1st edition. Belgium: Chandos Publishing; 2018.
Todeschini R, Baccini A. Handbook of Bibliometric Indicators: Quantitative Tools for Studying and Evaluating Research. 1st edition. Italy: Wiley-VCH Verlag GmbH & Co. KGaA; 2016.
Miao Z, Humphreys BD, McMahon AP, Kim J. Multi-omics integration in the age of million single-cell data. Nat Rev Nephrol. 2021; 17(11):710-724. doi: 10.1038/s41581-021-00463-x
Gao F, Huang K, Xing Y. Artificial Intelligence in Omics. Genomics Proteomics Bioinformatics. 2022; 20(5):811-813. doi: 10.1016/j.gpb.2023.01.002
Newman LA. Breast cancer screening in low and middle-income countries. Best Pract Res Clin Obstet Gynaecol. 2022; 83:15-23. doi: 10.1016/j.bpobgyn.2022.03.018
Anderson BO, Ilbawi AM, Fidarova E, Weiderpass E, Stevens L, Abdel-Wahab M, et al. The Global Breast Cancer Initiative: a strategic collaboration to strengthen health care for non-communicable diseases. Lancet Oncol. 2021; 22(5):578-581. doi: 10.1016/S1470-2045(21)00071-1
Horr C, Buechler SA. Breast Cancer Consensus Subtypes: A system for subtyping breast cancer tumors based on gene expression. NPJ Breast Cancer. 2021; 7(1):136. doi: 10.1038/s41523-021-00345-2
Bernstam EV, Shireman PK, Meric-Bernstam F, N Zozus M, Jiang X, Brimhall BB, et al. Artificial intelligence in clinical and translational science: Successes, challenges and opportunities. Clin Transl Sci. 2022; 15(2):309-321. doi: 10.1111/cts.13175
Zhang B, Shi H, Wang H. Machine Learning and AI in Cancer Prognosis, Prediction, and Treatment Selection: A Critical Approach. J Multidiscip Healthc. 2023; 16:1779-1791. doi: 10.2147/JMDH.S410301
Koh DM, Papanikolaou N, Bick U, Illing R, Kahn Jr. CE, Kalpathi-Cramer J, et al. Artificial intelligence and machine learning in cancer imaging. Commun Med. 2022; 2:133. doi: 10.1038/s43856-022-00199-0
You JS, Jones PA. Cancer genetics and epigenetics: two sides of the same coin? Cancer Cell. 2012 Jul 10;22(1):9-20. doi: 10.1016/j.ccr.2012.06.008
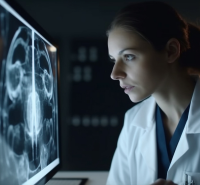
Downloads
Published
How to Cite
Issue
Section
License
Copyright (c) 2024 Revista de la Facultad de Medicina Humana

This work is licensed under a Creative Commons Attribution 4.0 International License.